The AI Will Not See You Now
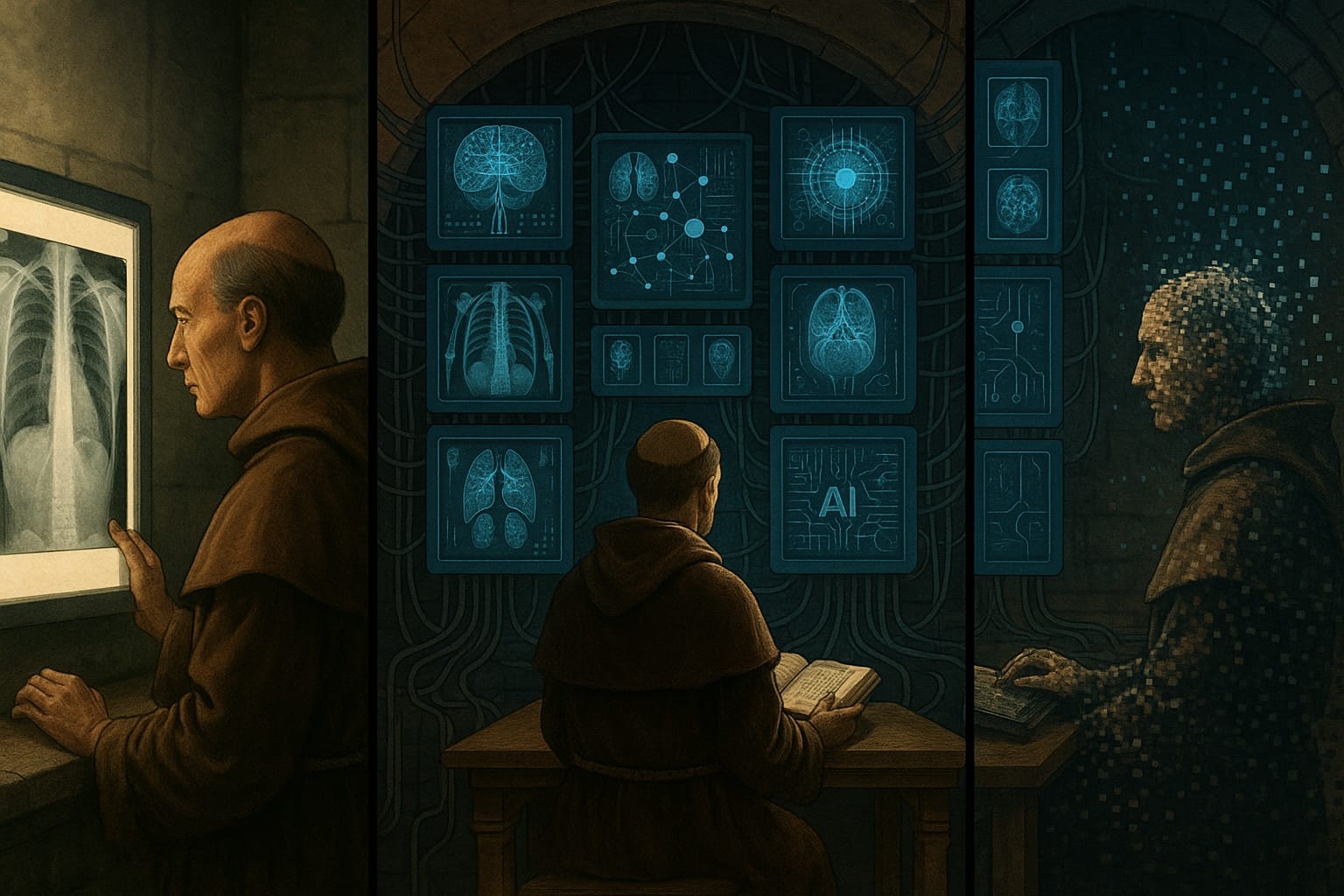
A radiologist warns that the art of medicine is vanishing—and AI won’t be there when we need it most.
By Ram Srinivasan, MD, PhD
As a radiologist, I’ve seen artificial intelligence transform my field from the inside out. What was once an apprenticeship of perception and pattern recognition—slowly built through years of practice—is now a proving ground for AI systems that promise faster, cheaper, and more consistent results than any human ever could. But radiology isn’t the endpoint of this transformation. It’s the prototype. And what’s happening here should serve as a warning to every corner of medicine.
We are not easing into an era of AI support. We are sprinting toward full automation—replacing judgment with prediction, training with efficiency, and human skill with machine inference. And unless we change course, we risk losing the entire art of medicine within a single generation.
This isn’t speculation. We’re already seeing it in other fields. Junior software developers are being passed over for hiring because AI tools like GitHub Copilot can produce boilerplate code in seconds. Entry-level graphic designers and copywriters are finding themselves displaced before they can learn the craft. When there’s no space for beginners, there’s no pipeline for mastery. And when the experts retire, no one is left.
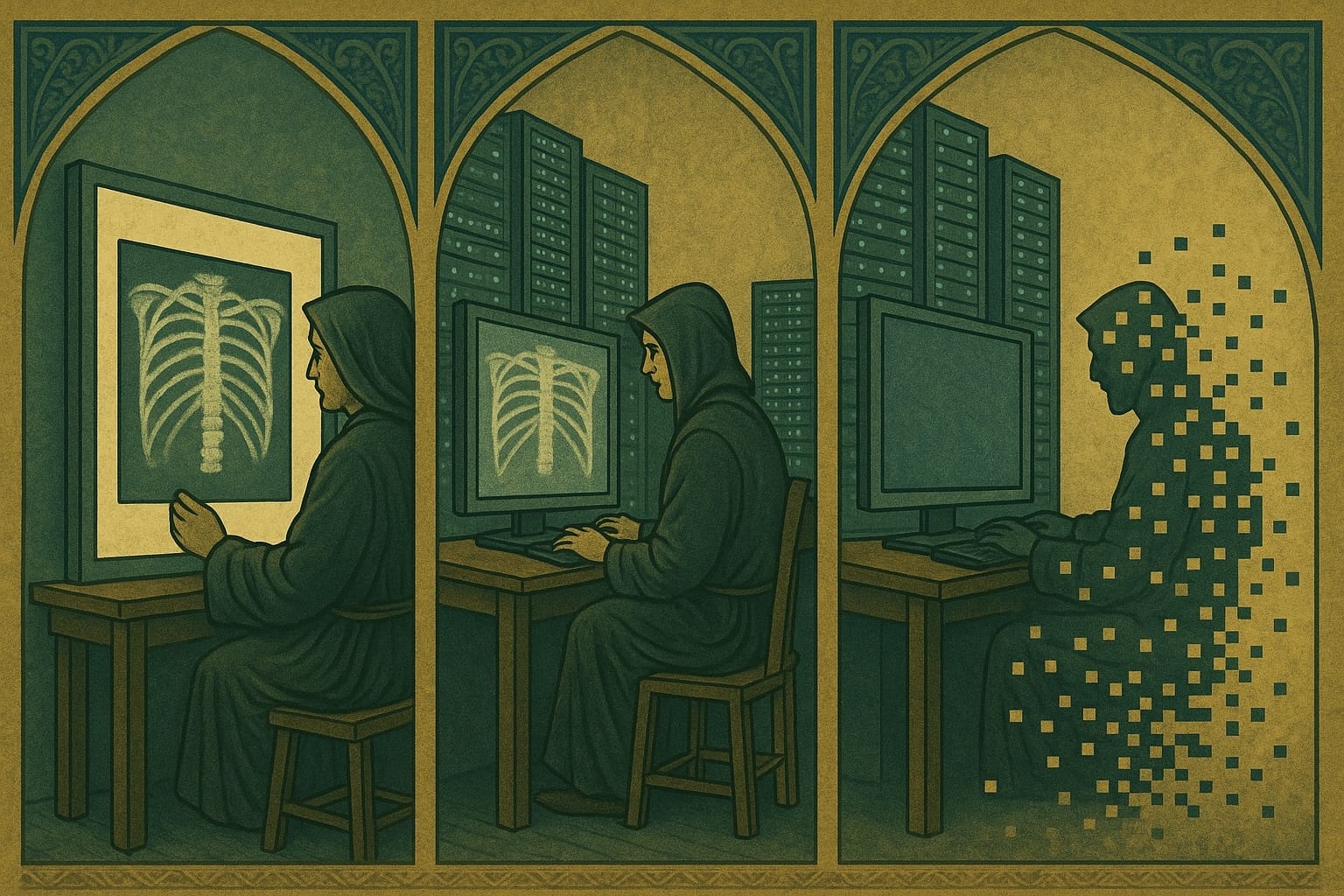
Medicine is heading the same way. As more workflows are automated—from scan interpretation to clinical decision support—fewer trainees will be given the chance to develop real, independent diagnostic skills. They will rely on the system to make the call, and in doing so, lose the capacity to be the system when it fails.
And it will fail.
In a world shaped by cyberwarfare, ransomware, data poisoning, and infrastructure breakdowns, the idea that AI systems are invulnerable is not just naïve—it’s reckless. When these tools go dark, the only thing that can keep care going is a human being with the knowledge and confidence to act without them.
But if that human has never been allowed to make the diagnosis themselves, or make the call without the machine whispering the answer, they won’t be a fallback—they’ll be another point of failure.
There’s a dangerous illusion spreading across medicine: that AI can do the work, and we’ll just have humans in the loop to supervise. It’s the Tesla self-driving fallacy dressed in a lab coat. But supervision without fluency is theater. It’s not safety. It’s submission.
Radiologists—and medical professionals more broadly—are the monks in this story. For generations, they have passed down knowledge through dedicated study, oral tradition, and guided practice. Now, the monastery is being filled with servers, and the monks are being asked to sign off on the work of algorithms, until the monks can be vacated entirely.
But one human overseeing a thousand AI-driven interpretations isn’t preservation—it’s institutional amnesia. Real expertise doesn’t survive through observation. It survives through use. Line by line. Scan by scan. If medicine is to endure, we must train humans to sustain healthcare delivery independently of AI—not alongside it, not supervising it, but beyond it when needed.
There’s a dangerous illusion spreading across medicine: that AI can do the work, and we’ll just have humans in the loop to supervise.
And this philosophy must extend beyond radiology. Every part of medicine—from prescribing to procedural care—faces the same risk: that cost-saving automation will erode the human core of clinical practice. And once that erosion starts, it accelerates. Hospitals, eager to cut labor costs, won’t just automate workflows—they’ll cut the humans who know how the system works. And then one day, the system will break, and we will find ourselves with no one who remembers how to fix it.
The future of medicine should absolutely include AI. But not as a replacement. As a partner. The only way to ensure that partnership works is to build a generation of clinicians who are not just AI-literate but AI-independent—trained to stand in when the infrastructure falls, to diagnose when the models falter, and to care when the machines go silent.
If we fail to protect that generational transfer of skill, the loss won’t just be technical or generational. It will be civilizational. A thousand years of accumulated judgment, pattern recognition, and clinical intuition—gone in a single upgrade cycle.
The tightrope we’re walking isn’t between human and machine. It’s between continuity and total system collapse.
About the Author
Ram Srinivasan, MD, PhD, is a practicing radiologist, engineer, and educator based in the Bay Area.